Smartphone Camera for Angiographic Computer Vision in Vascular Medicine
DOI:
https://doi.org/10.62487/82grqt38Keywords:
TensorFlow, Artery Disease, Smartphone Application, Machine Learning, Computer Vision, Artificial intelligenceAbstract
Aim: This study aimed to develop a TensorFlow Lite algorithm for angiography classification and to deploy it on a basic mobile smartphone device, thereby verifying the proof of concept for creating a comprehensive end-to-end mobile computer vision application for vascular medicine. Materials and Methods: After ethical approval by the local ethics committee, we collected institutional and open source peripheral angiograms of lower limbs. The angiograms were labeled by a researcher with more than 10 years of experience in vascular surgery. The labeling included dividing the angiograms according to their anatomical pattern into the Global Limb Anatomic Staging System (GLASS). The model was developed using the open-source TensorFlow framework for general image classification and deployed as an Android application. Results: The model utilized 700 angiograms, distributed as follows within the femoropoliteal GLASS disease (fp) categories: fp0 – 187 images, fp1 – 136 images, fp2 – 128 images, fp3 – 97 images, fp4 – 152 images. The reference dataset included 372 non-angiographic images (not_angio). Consequently, the entire model included 1,072 images. After training and deployment, the model demonstrated the following performance: a mean accuracy of 0.72. The best self-reported accuracy per class was for fp0 0.72, fp4 0.83 and not_angio 1.0 classes. Conclusion: We discovered that a smartphone camera could be utilized for angiographic computer vision through end-to-end applications accessible to every healthcare professional. However, the predictive abilities of the model are limited and require improvement. The development of a robust angiographic computer vision smartphone application should incorporate an upload function, undergo validation through head-to-head human-machine comparisons, potentially include segmentation, and feature a prospective design with explicit consent for using collected data in the development of AI models.
Angiographic Computer Vision:
FP GLASS
This is the web version of the angiographic computer vision tool for classifying femoropopliteal angiograms according to FP GLASS, as described in the article. For further details, please refer to the 'How to Use' section.
Instructions:
- Upload or capture an angiogram image.
- Crop the image to remove any artifacts.
- Click Make Prediction to begin analysis.
Privacy Notice:
All processing and calculations are performed on the client-side, ensuring complete data privacy with no data transferred to third-party servers.
Download and Retrain:
The underlying Machine Learning model can be downloaded and retrained with new data
here.
Original Article
Background
Image classification in vascular medicine:
Vascular medicine is the field of medicine with very high concentration of imaging. Vascular specialists routinely use imaging every day for diagnostics and treatment of their patients. Imaging is a modality where we must interpret visual information, which inherently involves some subjectivity and is limited by our current individual understanding of what we know. Computer vision could standardize the interpretation of the information we visualize. It allows us to obtain a second, maximally independent, and at the same time, evidence-based opinion that could guide complex clinical decisions.
Most of the available studies in vascular medicine are currently focused on the analysis of Doppler waveforms1 2 3 4, ultrasound images5, and peripheral angiograms6 7 8, magnetic resonance and computed tomography images9 10. The models available today are primarily experimental in nature and find application in clinical practice only as laboratory or commercial tools. "Diamond open access"11 and end-to-end mobile applications for computer vision, which could be applied by medical specialists across the field of vascular medicine, are currently not available.
TensorFlow Lite
TensorFlow Lite is a component of the TensorFlow image classification platform that offers the capability to deploy custom-made and optimized pre-trained models as mobile applications on smartphones12 13.
Aim
This study aimed to develop a TensorFlow Lite algorithm for angiography classification and deploy it on a basic mobile smartphone device, thereby verifying the proof of concept for creating a comprehensive end-to-end mobile computer vision application for vascular medicine.
Material and Methods
Data Collection
Our dataset comprised two subsets. The first subset included images collected through a web-indexed search and previously used for automated machine learning (AutoML) model development in our prior research6. The second subset was gathered through institutional channels following ethical approval of our study: Ethic Committee University Leipzig Medical Faculty, Protocol Nr. 333/23-ek from 24.10.2023.
The images consisted of digital subtraction angiograms (DSA) of the femoral, popliteal, and femoropopliteal segments of the lower limb arteries. These images were labeled according to the most applicable grade of femoropopliteal disease using the Global Limb Anatomic Staging System (GLASS)14, as described in our previous research6. Additionally, we created a random reference set of non-angiographic images from the Kaggle platform15. Thus, our entire dataset included six subsets: Grades 0-4 of femoropopliteal disease according to GLASS, and a "non-angio" subset.
Model training, deployment, and extraction
We employed the most straightforward method of TensorFlow Lite model development using the Teachable Machine platform and a standard color image model with an input resizing of 224x224 pixels. The labeled data were trained with the following settings: 10 epochs, a batch size of 16, and a learning rate of 0.001, standard data split. The model's performance was assessed using a confusion matrix and accuracy per class. After deployment, the model was extracted as a quantized TensorFlow Lite file.
Application Deployment Using TensorFlow Lite Model with Android Studio
We utilized the standard TensorFlow Lite example model from the GitHub repository for image classification on the Android system16. The model was extracted from the repository and modified within Android Studio Flamingo (2022.2.1 Patch2) by adding the previously created TensorFlow Lite quantized model into the assets category, as shown in Figure 1.
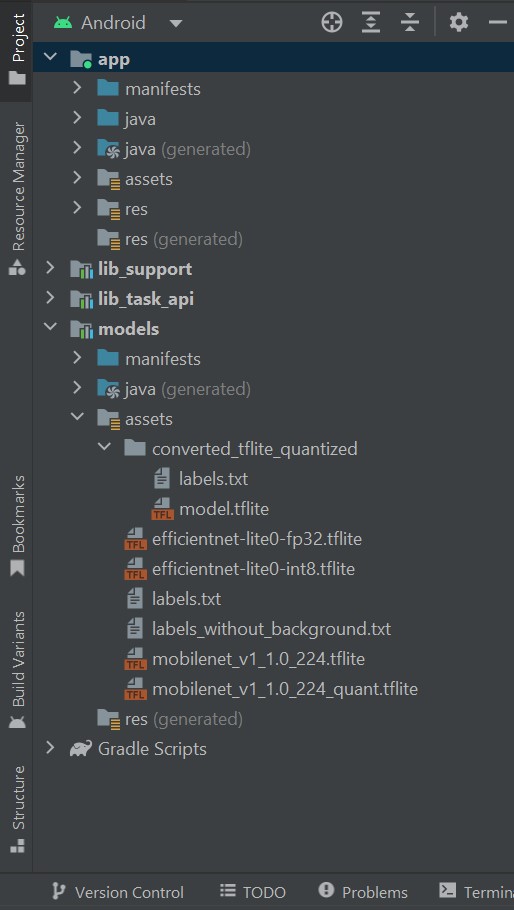
Figure 1: Incorporation of TensorFlow Lite quantized model into the assets category
APK Deployment and Installation on a Smartphone
After incorporating our customized model, we customized the TensorFlow Lite application by adding a unique name, "ML GLASS," and an icon and deployed on an Android Smartphone Sony Xperia XQ-AS52 Android 12, as shown in Figure 2.
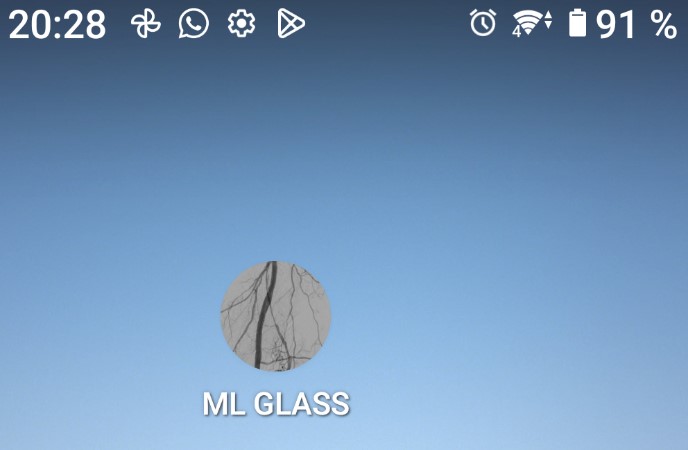
Figure 2: Installed APK on the smartphone
Testing with Unknown Angiograms
After deployment, the application was used to identify previously unknown random static angiograms using a smartphone camera with the following presets: Standard frame: 640x480, Crop: 224x224, View: 480x480, Rotation: 90 degrees, Threads: 9, Model: Quantized_MobileNet, Device: CPU.
Results
Dataset
We analyzed a total of 700 angiograms, distributed as follows within the femoropoliteal GLASS disease (fp) categories: fp0 – 187 images, fp1 – 136 images, fp2 – 128 images, fp3 – 97 images, fp4 – 152 images. The reference dataset included 372 non-angiographic images (not_angio). Consequently, the entire model included 1,072 images.
Performance
After training and deployment, the model demonstrated the following performance: a mean accuracy of 0.72, accuracy per class (Figure 3), the confusion matrix (Figure 4), and accuracy per epoch (Figure 5).
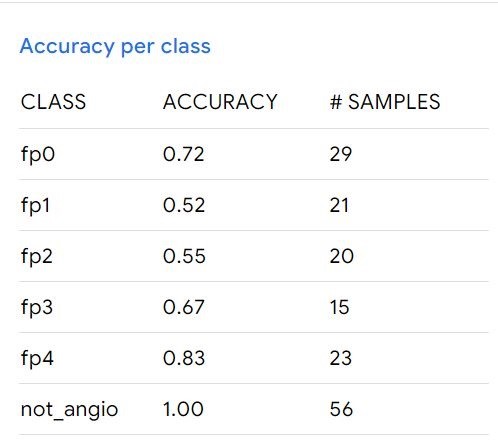
Figure 3: Accuracy of the model per class
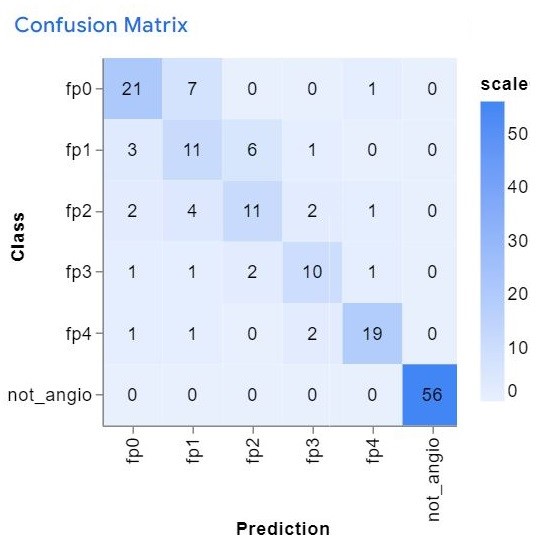
Figure 4: Confusion Matrix: demonstrating the model's performance.
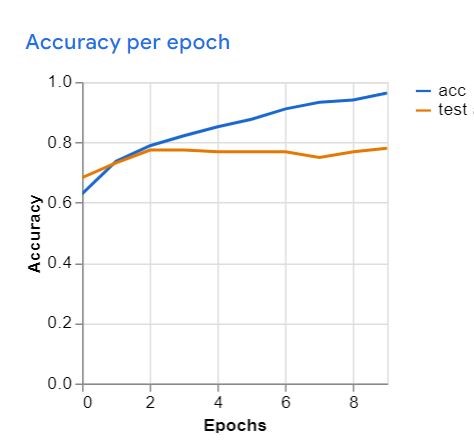
Figure 5: Model accuracy by epoch during training
The model's performance was also assessed with previously unseen angiograms. The results are illustrated in Figures 6, 7. A sufficient head-to-head Kappa comparison with an independent human researcher was not possible because the model's predictive values varied depending on external light, image position on the monitor, and movement of the smartphone. However, the model was able to correctly recognize the angiograms within the provided accuracy, as shown in Figures 6, 7.
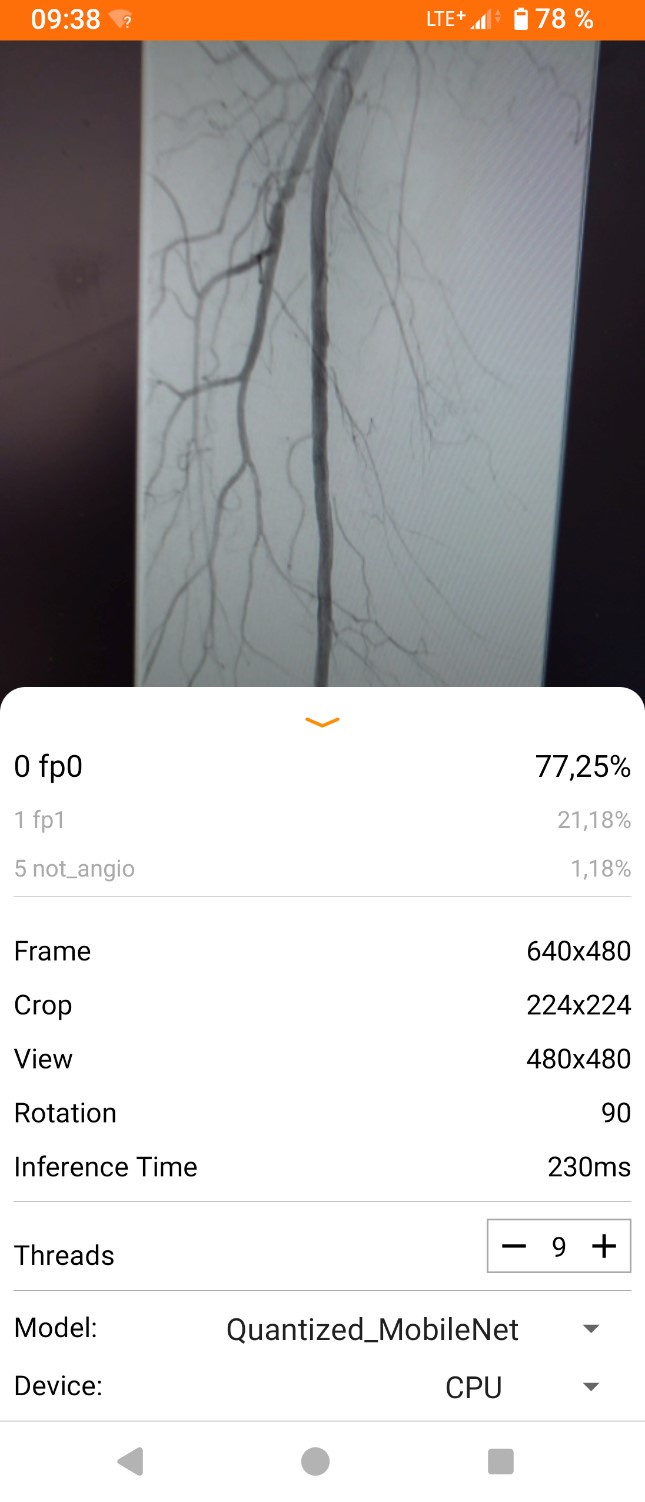
Figure 6: Screenshot of working application.
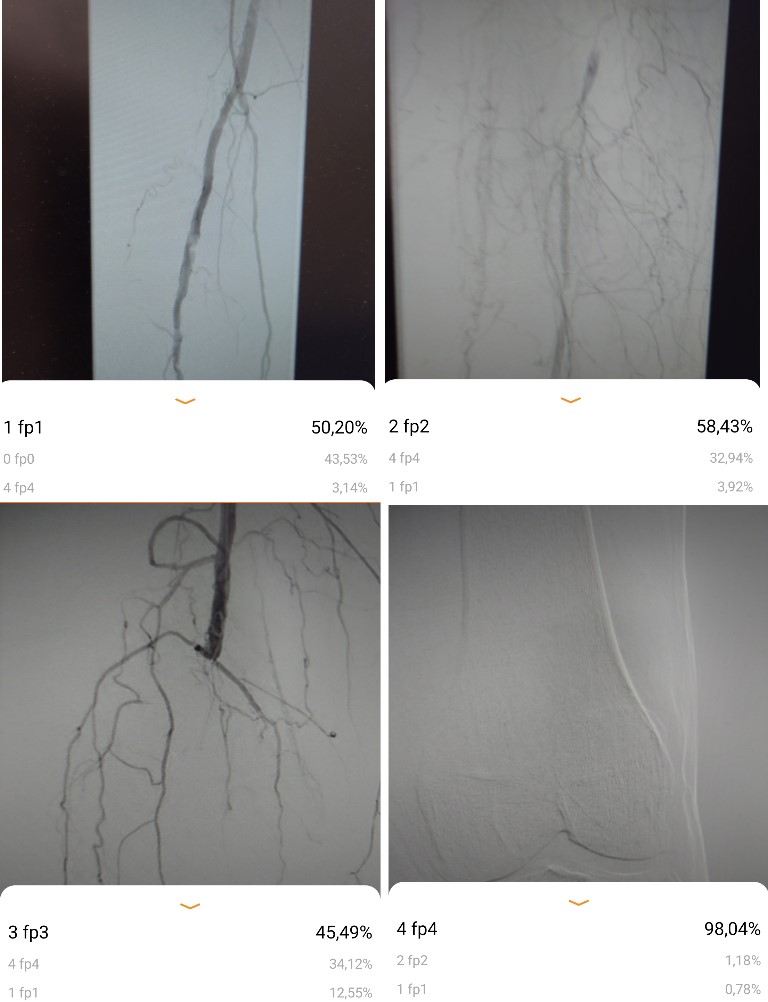
Figure 7: Screenshots of disease classification according to the most applicable grade of femoropoliteal disease in GLASS
Discussion
Practical standpoint
This research validated the concept of creating a mobile application for angiogram classification using computer vision on a smartphone camera. The deployment is feasible on essentially any Android smartphone. The application was able to perfectly distinguish between angiographic and non-angiographic images with an accuracy of 1.0. Good results were also observed for diseases categorized as fp0 and fp4 due to the definitive disease patterns. However, lower performance was noted for fp1 and fp2. The model's predictive abilities were influenced by external factors such as external light and camera movement, which limit its application within healthcare settings. Nonetheless, it has the potential to be used by non-vascular specialists and vascular trainees as a supportive tool.
Limitations
The main limitation of this research is the application of a holistic image classification algorithm for our computer vision model. Segmentation could potentially outperform our algorithm and yield better performance. However, the primary goal of this study was not to build a powerful model but to prove the concept of developing a mobile application for computer vision in vascular medicine.
The second limitation is that the camera was used in movie mode; therefore, the predictive value changes with angulation or light exposure. For example, the upload function for screenshots could provide a constant predictive value and improve the model.
The third limitation is the absence of a head-to-head human-machine comparison, due to the proof-of-concept study design and the challenging interpretation of results caused by the camera's movie mode, as mentioned in the second limitation.
Another limitation of our research is its retrospective nature. Therefore, the patient data used and consents obtained were only for general research purposes, not specifically for the development of an artificial intelligence (AI) model. As a result, our current model will remain an experimental project. The development of a powerful angiographic computer vision smartphone application should incorporate an upload function, be proven in head-to-head human-machine comparisons, potentially include segmentation, and feature a prospective design with explicit consent for using collected data in the development of AI models17.
To the best of our knowledge, this is the first study to explore the development of an end-to-end smartphone application for angiographic computer vision in vascular medicine.
Conclusion
We discovered that a smartphone camera could be utilized for angiographic computer vision through end-to-end applications accessible to every healthcare professional. However, the predictive abilities of the model are limited and require improvement. The development of a robust angiographic computer vision smartphone application should incorporate an upload function, undergo validation through head-to-head human-machine comparisons, potentially include segmentation, and feature a prospective design with explicit consent for using collected data in the development of AI models.
Conflict of Interest: Authors state that no conflict of interest exists.
Authorship: YR: Concept, data analysis, original draft, survey. YR, VR, MD: Review and editing.
The Web3 rewards were donated to water.org by the corresponding author.
References
1 Kim S, Hahn J-O, Youn BD. Detection and Severity Assessment of Peripheral Occlusive Artery Disease via Deep Learning Analysis of Arterial Pulse Waveforms: Proof-of-Concept and Potential Challenges. Front Bioeng Biotechnol. 2020;8:720. doi:10.3389/fbioe.2020.00720.
2 McBane RD, Murphree DH, Liedl D, et al. Artificial intelligence for the evaluation of peripheral artery disease using arterial Doppler waveforms to predict abnormal ankle-brachial index. Vasc Med. 2022;27(4):333-342. doi:10.1177/1358863X221094082.
3 Normahani P, Sounderajah V, Mandic D, Jaffer U. Machine learning-based classification of arterial spectral waveforms for the diagnosis of peripheral artery disease in the context of diabetes: A proof-of-concept study. Vasc Med. 2022;27(5):450-456. doi:10.1177/1358863X221105113.
4 Masoumi Shahrbabak S, Kim S, Youn BD, et al. Peripheral artery disease diagnosis based on deep learning-enabled analysis of non-invasive arterial pulse waveforms. Comput Biol Med. 2024;168:107813. doi:10.1016/j.compbiomed.2023.107813.
5 Kordzadeh A, Askari A, Abbassi OA, Sanoudos N, Mohaghegh V, Shirvani H. Artificial intelligence and duplex ultrasound for detection of carotid artery disease. Vascular. 2023;31(6):1187-1193. doi:10.1177/17085381221107465.
6 Rusinovich Y, Rusinovich V, Buhayenka A, et al. Classification of anatomic patterns of peripheral artery disease with automated machine learning (AutoML). Vascular. 2024:17085381241236571. doi:10.1177/17085381241236571.
7 Ou C, Qian Y, Chong W, et al. A deep learning-based automatic system for intracranial aneurysms diagnosis on three-dimensional digital subtraction angiographic images. Med Phys. 2022;49(11):7038-7053. doi:10.1002/mp.15846.
8 Irfan M, Malik KM, Ahmad J, Malik G. StrokeNet: An automated approach for segmentation and rupture risk prediction of intracranial aneurysm. Comput Med Imaging Graph. 2023;108:102271. doi:10.1016/j.compmedimag.2023.102271.
9 Koç U, Sezer EA, Özkaya YA, et al. Elevating healthcare through artificial intelligence: analyzing the abdominal emergencies data set (TR_ABDOMEN_RAD_EMERGENCY) at TEKNOFEST-2022. Eur Radiol. 2023. doi:10.1007/s00330-023-10391-y.
10 Courtman M, Kim D, Wit H, et al. Deep Learning Detection of Aneurysm Clips for Magnetic Resonance Imaging Safety. J Imaging Inform Med. 2024;37(1):72-80. doi:10.1007/s10278-023-00932-8.
11 Wikipedia. Diamond open access. link. Accessed March 21, 2024.
12 TensorFlow. Image classification. link. Updated 2023. Accessed March 11, 2024.
13 TensorFlow. Image classification with TensorFlow Lite Model Maker. link. Updated 2023. Accessed March 11, 2024.
14 Conte MS, Bradbury AW, Kolh P, et al. Global Vascular Guidelines on the Management of Chronic Limb-Threatening Ischemia. Eur J Vasc Endovasc Surg. 2019;58(1S):S1-S109.e33. doi:10.1016/j.ejvs.2019.05.006.
15 Kaggle. Dataset. link. Accessed 2023.
16 Github. Tensorflow. Examples. Image classification. Android. link. Accessed March 12, 2024.
17 Rusinovich Y. Human-centered Evaluation of AI and ML Projects. Web3MLHS. 2024;1(2). doi:10.62487/ypqhkt57.
References
Kim S, Hahn J-O, Youn BD. Detection and Severity Assessment of Peripheral Occlusive Artery Disease via Deep Learning Analysis of Arterial Pulse Waveforms: Proof-of-Concept and Potential Challenges. Front Bioeng Biotechnol. 2020;8:720. doi:10.3389/fbioe.2020.00720. DOI: https://doi.org/10.3389/fbioe.2020.00720
McBane RD, Murphree DH, Liedl D, et al. Artificial intelligence for the evaluation of peripheral artery disease using arterial Doppler waveforms to predict abnormal ankle-brachial index. Vasc Med. 2022;27(4):333-342. doi:10.1177/1358863X221094082. DOI: https://doi.org/10.1177/1358863X221094082
Normahani P, Sounderajah V, Mandic D, Jaffer U. Machine learning-based classification of arterial spectral waveforms for the diagnosis of peripheral artery disease in the context of diabetes: A proof-of-concept study. Vasc Med. 2022;27(5):450-456. doi:10.1177/1358863X221105113. DOI: https://doi.org/10.1177/1358863X221105113
Masoumi Shahrbabak S, Kim S, Youn BD, et al. Peripheral artery disease diagnosis based on deep learning-enabled analysis of non-invasive arterial pulse waveforms. Comput Biol Med. 2024;168:107813. doi:10.1016/j.compbiomed.2023.107813. DOI: https://doi.org/10.1016/j.compbiomed.2023.107813
Kordzadeh A, Askari A, Abbassi OA, Sanoudos N, Mohaghegh V, Shirvani H. Artificial intelligence and duplex ultrasound for detection of carotid artery disease. Vascular. 2023;31(6):1187-1193. doi:10.1177/17085381221107465. DOI: https://doi.org/10.1177/17085381221107465
Rusinovich Y, Rusinovich V, Buhayenka A, et al. Classification of anatomic patterns of peripheral artery disease with automated machine learning (AutoML). Vascular. 2024:17085381241236571. doi:10.1177/17085381241236571. DOI: https://doi.org/10.1177/17085381241236571
Ou C, Qian Y, Chong W, et al. A deep learning-based automatic system for intracranial aneurysms diagnosis on three-dimensional digital subtraction angiographic images. Med Phys. 2022;49(11):7038-7053. doi:10.1002/mp.15846. DOI: https://doi.org/10.1002/mp.15846
Irfan M, Malik KM, Ahmad J, Malik G. StrokeNet: An automated approach for segmentation and rupture risk prediction of intracranial aneurysm. Comput Med Imaging Graph. 2023;108:102271. doi:10.1016/j.compmedimag.2023.102271. DOI: https://doi.org/10.1016/j.compmedimag.2023.102271
Koç U, Sezer EA, Özkaya YA, et al. Elevating healthcare through artificial intelligence: analyzing the abdominal emergencies data set (TR_ABDOMEN_RAD_EMERGENCY) at TEKNOFEST-2022. Eur Radiol. 2023. doi:10.1007/s00330-023-10391-y. DOI: https://doi.org/10.1007/s00330-023-10391-y
Courtman M, Kim D, Wit H, et al. Deep Learning Detection of Aneurysm Clips for Magnetic Resonance Imaging Safety. J Imaging Inform Med. 2024;37(1):72-80. doi:10.1007/s10278-023-00932-8. DOI: https://doi.org/10.1007/s10278-023-00932-8
Wikipedia. Diamond open access. https://en.wikipedia.org/wiki/Diamond_open_access. Accessed March 21, 2024.
TensorFlow. Image classification. https://www.tensorflow.org/lite/models/modify/model_maker/image_classification. Updated 2023. Accessed March 11, 2024.
TensorFlow. Image classification with TensorFlow Lite Model Maker. https://www.tensorflow.org/lite/models/modify/model_maker/image_classification. Updated 2023. Accessed March 11, 2024.
Conte MS, Bradbury AW, Kolh P, et al. Global Vascular Guidelines on the Management of Chronic Limb-Threatening Ischemia. Eur J Vasc Endovasc Surg. 2019;58(1S):S1-S109.e33. doi:10.1016/j.ejvs.2019.05.006. DOI: https://doi.org/10.1016/j.ejvs.2019.05.006
Kaggle. Dataset. https://www.kaggle.com/datasets?tags=13207-Computer+Vision. Accessed 2023.
Github. Tensorflow. Examples. Image classification. Android. https://github.com/tensorflow/examples/tree/master/lite/examples/image_classification/android. Accessed March 12, 2024.
Rusinovich Y. Human-centered Evaluation of AI and ML Projects. Web3MLHS. 2024;1(2). doi:10.62487/ypqhkt57. DOI: https://doi.org/10.62487/ypqhkt57
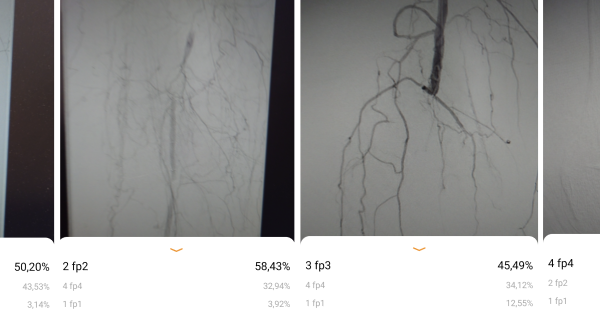
Downloads
Published
Issue
Section
License
Copyright (c) 2024 Yury Rusinovich, Volha Rusinovich, Markus Doss (Author)

This work is licensed under a Creative Commons Attribution-ShareAlike 4.0 International License.
Add a Comment:
Comments:
Article views: 0