Human-centered Evaluation of AI and ML Projects
DOI:
https://doi.org/10.62487/ypqhkt57Keywords:
Evaluation of AI, Human-centered AIAbstract
With this editorial, we inaugurate the next issue of our journal, which is dedicated to showcasing AI, ML, and E-Health projects within real healthcare environments.
Interested in promoting your brand or product here? Contact us at
info@mhs.ink
Editorial
With this editorial, we inaugurate the next issue of our journal, which is dedicated to showcasing AI, ML and E-Health models within real healthcare environments. We cordially invite authors to submit their works for publication. Each submission will undergo a rigorous peer review, with a special focus on the human-centered aspects of the proposed original project. The evaluation process will adhere to evidence-based guidelines continuously refined by our ongoing web research and the insights published in our foundational issue, "Why ML in Health Science." Published original projects will be recognized with our Blockchain Token, MLHS, and added to the repository “Web3 Certificate: Human-Centered Project”1.
It's important to clarify that our recommendations are not intended to replace the guidance of official regulatory bodies. Rather, they are designed to enhance the integration of human-centered considerations in AI and ML projects, thereby promoting sustainable human-AI collaboration.
Within this editorial, you will find our current recommendations, each substantiated by research or endorsements from official regulatory authorities. These recommendations will be utilized for the peer-review process:
1. Maintain human oversight in machine-to-machine interactions, ensuring that critical decisions involve human judgment and accountability2 3 4 5 6 7.
2. Provide transparent information about the developer team, such as profiles on social networks (LinkedIn, X, etc.)6 8 9.
3. Ensure transparency regarding the algorithms of the models6 8 9 10 11 12.
4. Build your model on existing and proven data13 14 15.
5. Regularly consult an independent human expert to validate the stability of your AI/ML system. Employ robust validation methods to compare human and machine decisions, ensuring continuous accuracy and fairness5 2 3 8 16 17 9 10 11.
6. Inform the end-users of your model, such as patients or clients, about the utilization of AI/ML in communication, including diagnostics and treatment processes18 19 20 21 8 7 22 12.
7. Inform the users of your model, such as patients or clients, about the utilization of their data for training of your model, if such training is performed18 19 20 21 22 12.
8. Implement a feedback system to collect insights from your end users, such as patients or clients, regarding the performance and impact of your model20 21 8 12.
9. Guarantee that your project adheres to the prevailing guidelines and standards in Health Science and Healthcare, ensuring compliance, safety, and efficacy in all applications2 12.
10. Avoid using confounders that could lead to social scoring and categorization of humans, such as nationality, race, immigration status, or religion3 2 23 24 25 26 27 28 29 30 31 32 33 34 35 8 36 37.
11. Provide Diamond Open Access to at least the beta version of your project, meaning no fees are charged38.
12. Incorporate team members with medical backgrounds who have regular interactions with real patients into your project2 7 12.
13. Prominently highlight a “Human-centered” approach in your White Paper, website, and social media posts, underscoring the commitment to prioritizing human well-being and ethical standards in your projects39.
14. Engage in charitable activities or make donations (e.g., to organizations like UNICEF, Water.org, etc.)40 41 42 43.
Conflict of Interest: no conflict of interest exists.
References
1 ML in Health Science. Repository "Web3 Certificate: Human-Centered Project." link. Accessed March 31, 2024.
2 World Health Organisation, ed. Ethics and governance of artificial intelligence for health. Guidance on large multi-modal models; 2024. link.
3 European Parliamentary Research Service. Artificial intelligence act. link.
4 European Parliamentary Research Service. Metaverse. Opportunities, risks and policy implications. link. Accessed March 10, 2024.
5 Nichol AA, Sankar PL, Halley MC, Federico CA, Cho MK. Developer Perspectives on Potential Harms of Machine Learning Predictive Analytics in Health Care: Qualitative Analysis. J Med Internet Res. 2023;25:e47609. doi:10.2196/47609.
6 Sartor G. The impact of the General Data Protection Regulation (GDPR) on artificial intelligence: Study. Brussels: European Parliament; 2020. link.
7 Rusinovich Y, Rusinovich A, Rusinovich V. Do You Agree with AI Making Decisions About Your Treatment? A Comparative Survey of IT and Healthcare Practitioners. Web3MLHS. 2024;1(1). doi:10.62487/m67cnn54.
8 National Telecommunications and Information Administration. AI Accountability Policy Report. link. Accessed April 12, 2024.
9 Collins GS, Reitsma JB, Altman DG, Moons KGM. Transparent reporting of a multivariable prediction model for individual prognosis or diagnosis (TRIPOD): the TRIPOD statement. BMJ. 2015;350:g7594. doi:10.1136/bmj.g7594.
10 Mongan J, Moy L, Kahn CE. Checklist for Artificial Intelligence in Medical Imaging (CLAIM): A Guide for Authors and Reviewers. Radiol Artif Intell. 2020;2(2):e200029. doi:10.1148/ryai.2020200029.
11 Liu X, Cruz Rivera S, Moher D, Calvert MJ, Denniston AK. Reporting guidelines for clinical trial reports for interventions involving artificial intelligence: the CONSORT-AI extension. Nat Med. 2020;26(9):1364-1374. doi:10.1038/s41591-020-1034-x.
12 Lekadir K, Osuala R, Gallin C, et al. FUTURE-AI: Guiding Principles and Consensus Recommendations for Trustworthy Artificial Intelligence in Medical Imaging; 2021. link.
13 Wagner MW, Ertl-Wagner BB. Accuracy of Information and References Using ChatGPT-3 for Retrieval of Clinical Radiological Information. Can Assoc Radiol J. 2024;75(1):69-73. doi:10.1177/08465371231171125.
14 Sharun K, Banu SA, Pawde AM, et al. ChatGPT and artificial hallucinations in stem cell research: assessing the accuracy of generated references - a preliminary study. Ann Med Surg (Lond). 2023;85(10):5275-5278. doi:10.1097/MS9.0000000000001228.
15 Athaluri SA, Manthena SV, Kesapragada VSRKM, Yarlagadda V, Dave T, Duddumpudi RTS. Exploring the Boundaries of Reality: Investigating the Phenomenon of Artificial Intelligence Hallucination in Scientific Writing Through ChatGPT References. Cureus. 2023;15(4):e37432. doi:10.7759/cureus.37432.
16 Antoniou T, Mamdani M. Evaluation of machine learning solutions in medicine. CMAJ. 2021;193(36):E1425-E1429. doi:10.1503/cmaj.210036.
17 Kwong JCC, Khondker A, Lajkosz K, et al. APPRAISE-AI Tool for Quantitative Evaluation of AI Studies for Clinical Decision Support. JAMA Netw Open. 2023;6(9):e2335377. doi:10.1001/jamanetworkopen.2023.35377.
18 Aggarwal R, Farag S, Martin G, Ashrafian H, Darzi A. Patient Perceptions on Data Sharing and Applying Artificial Intelligence to Health Care Data: Cross-sectional Survey. J Med Internet Res. 2021;23(8):e26162. doi:10.2196/26162.
19 Moorcraft SY, Marriott C, Peckitt C, et al. Patients' willingness to participate in clinical trials and their views on aspects of cancer research: results of a prospective patient survey. Trials. 2016;17:17. doi:10.1186/s13063-015-1105-3.
20 Parry MW, Markowitz JS, Nordberg CM, Patel A, Bronson WH, DelSole EM. Patient Perspectives on Artificial Intelligence in Healthcare Decision Making: A Multi-Center Comparative Study. Indian J Orthop. 2023;57(5):653-665. doi:10.1007/s43465-023-00845-2.
21 Richardson JP, Smith C, Curtis S, et al. Patient apprehensions about the use of artificial intelligence in healthcare. NPJ Digit Med. 2021;4(1):140. doi:10.1038/s41746-021-00509-1.
22 Rusinovich Y, Rusinovich V. Do You Consent to the Use of Your Biological Data for Training ML and AI Models? Online Survey Targeting Clinicians and Researchers. Web3MLHS. 2024;1(1). doi:10.62487/yyx99243.
23 Agency for Healthcare Research and Quality. Impact of Healthcare Algorithms on Racial and Ethnic Disparities in Health and Healthcare. link.
24 Schlundt DG, Franklin MD, Patel K, et al. Religious affiliation, health behaviors and outcomes: Nashville REACH 2010. Am J Health Behav. 2008;32(6):714-724. doi:10.5555/ajhb.2008.32.6.714.
25 Cary MP, Zink A, Wei S, et al. Mitigating Racial And Ethnic Bias And Advancing Health Equity In Clinical Algorithms: A Scoping Review. Health Aff (Millwood). 2023;42(10):1359-1368. doi:10.1377/hlthaff.2023.00553.
26 Shanklin R, Samorani M, Harris S, Santoro MA. Ethical Redress of Racial Inequities in AI: Lessons from Decoupling Machine Learning from Optimization in Medical Appointment Scheduling. Philos Technol. 2022;35(4):96. doi:10.1007/s13347-022-00590-8.
27 Allen A, Mataraso S, Siefkas A, et al. A Racially Unbiased, Machine Learning Approach to Prediction of Mortality: Algorithm Development Study. JMIR Public Health Surveill. 2020;6(4):e22400. doi:10.2196/22400.
28 Andrade C. Confounding. Indian J Psychiatry. 2007;49(2):129-131. doi:10.4103/0019-5545.33263.
29 Alsubaie MK, Dolezal M, Sheikh IS, et al. Religious coping, perceived discrimination, and posttraumatic growth in an international sample of forcibly displaced Muslims. Ment Health Relig Cult. 2021;24(9):976-992. doi:10.1080/13674676.2021.1973978.
30 Scheitle CP, Frost J, Ecklund EH. The Association between Religious Discrimination and Health: Disaggregating by Types of Discrimination Experiences, Religious Tradition, and Forms of Health. Scientific Study of Religion. 2023;62(4):845-868. doi:10.1111/jssr.12871.
31 Jordanova V, Crawford MJ, McManus S, Bebbington P, Brugha T. Religious discrimination and common mental disorders in England: a nationally representative population-based study. Soc Psychiatry Psychiatr Epidemiol. 2015;50(11):1723-1729. doi:10.1007/s00127-015-1110-6.
32 Baqai B, Azam L, Davila O, Murrar S, Padela AI. Religious Identity Discrimination in the Physician Workforce: Insights from Two National Studies of Muslim Clinicians in the US. J Gen Intern Med. 2023;38(5):1167-1174. doi:10.1007/s11606-022-07923-5.
33 Bender M, van Osch Y, He J, Güngör D, Eldja A. The role of perceived discrimination in linking religious practices and well-being: A study among Muslim Afghan refugees in the Netherlands. Int J Psychol. 2022;57(4):445-455. doi:10.1002/ijop.12854.
34 Wu Z, Schimmele CM. Perceived religious discrimination and mental health. Ethn Health. 2021;26(7):963-980. doi:10.1080/13557858.2019.1620176.
35 Sharif MZ, Truong M, Alam O, et al. The association between experiences of religious discrimination, social-emotional and sleep outcomes among youth in Australia. SSM Popul Health. 2021;15:100883. doi:10.1016/j.ssmph.2021.100883.
36 Rusinovich Y, Rusinovich V. Confounders in Predictive Medical Models: Roles of Nationality and Immigrant Status. Web3MLHS. 2024;1(1). doi:10.62487/vc54ms96.
37 Rusinovich Y, Rusinovich V. Confounders in Predictive Medical Models: The Role of Religion. Web3MLHS. 2024;1(1). doi:10.62487/2rm68r13.
38 An introduction to the UNESCO Recommendation on Open Science; 2022. link.
39 Shneiderman B. Human-Centered AI. Oxford University Press; 2022. link.
40 Zare H, Eisenberg M, Anderson G. Charity Care and Community Benefit in Non-Profit Hospitals: Definition and Requirements. Inquiry. 2021;58:469580211028180. doi:10.1177/00469580211028180.
41 Schlesinger M, Quon N, Wynia M, Cummins D, Gray B. Profit-seeking, corporate control, and the trustworthiness of health care organizations: assessments of health plan performance by their affiliated physicians. Health Serv Res. 2005;40(3):605-645. doi:10.1111/j.1475-6773.2005.00377.x.
42 Tu HT, Reschovsky JD. Assessments of medical care by enrollees in for-profit and nonprofit health maintenance organizations. N Engl J Med. 2002;346(17):1288-1293. doi:10.1056/NEJMsa011250.
43 Gholamzadeh Nikjoo R, Partovi Y, Joudyian N. Involvement of charities in Iran's health care system: a qualitative study on problems and executive/legal/supportive requirements. BMC Health Serv Res. 2021;21(1):181. doi:10.1186/s12913-021-06187-9.
References
ML in Health Science. Repository "Web3 Certificate: Human-Centered Project". https://mlhs.ink/Certificate/. Accessed March 31, 2024.
European Parliamentary Research Service. Metaverse. Opportunities, risks and policy implications. https://www.europarl.europa.eu/cmsdata/268589/eprs-briefing-metaverse_EN.pdf. Accessed March 10, 2024.
World Health Organisation, ed. Ethics and governance of artificial intelligence for health. Guidance on large multi-modal models; 2024.
European Parliamentary Research Service. Artificial intelligence act. https://www.europarl.europa.eu/RegData/etudes/BRIE/2021/698792/EPRS_BRI(2021)698792_EN.pdf.
Nichol AA, Sankar PL, Halley MC, Federico CA, Cho MK. Developer Perspectives on Potential Harms of Machine Learning Predictive Analytics in Health Care: Qualitative Analysis. J Med Internet Res. 2023;25:e47609. doi:10.2196/47609. DOI: https://doi.org/10.2196/47609
Sartor G. The impact of the General Data Protection Regulation (GDPR) on artificial intelligence: Study. Brussels: European Parliament; 2020.
Rusinovich Y, Rusinovich A, Rusinovich V. Do You Agree with AI Making Decisions About Your Treatment? A Comparative Survey of IT and Healthcare Practitioners. Web3MLHS. 2024;1(1). doi:10.62487/m67cnn54. DOI: https://doi.org/10.62487/m67cnn54
National Telecommunications and Information Administration. AI Accountability Policy Report. https://www.ntia.gov/sites/default/files/publications/ntia-ai-report-final.pdf. Accessed April 12, 2024.
Collins GS, Reitsma JB, Altman DG, Moons KGM. Transparent reporting of a multivariable prediction model for individual prognosis or diagnosis (TRIPOD): the TRIPOD statement. BMJ. 2015;350:g7594. doi:10.1136/bmj.g7594. DOI: https://doi.org/10.1136/bmj.g7594
Lekadir K, Osuala R, Gallin C, et al. FUTURE-AI: Guiding Principles and Consensus Recommendations for Trustworthy Artificial Intelligence in Medical Imaging; 2021.
Liu X, Cruz Rivera S, Moher D, Calvert MJ, Denniston AK. Reporting guidelines for clinical trial reports for interventions involving artificial intelligence: the CON-SORT-AI extension. Nat Med. 2020;26(9):1364-1374. doi:10.1038/s41591-020-1034-x. DOI: https://doi.org/10.1136/bmj.m3164
Mongan J, Moy L, Kahn CE. Checklist for Artificial Intelligence in Medical Imaging (CLAIM): A Guide for Authors and Reviewers. Radiol Artif Intell. 2020;2(2):e200029. doi:10.1148/ryai.2020200029. DOI: https://doi.org/10.1148/ryai.2020200029
Wagner MW, Ertl-Wagner BB. Accuracy of Information and References Using ChatGPT-3 for Retrieval of Clinical Radiological Information. Can Assoc Radiol J. 2024;75(1):69-73. doi:10.1177/08465371231171125. DOI: https://doi.org/10.1177/08465371231171125
Sharun K, Banu SA, Pawde AM, et al. ChatGPT and artificial hallucinations in stem cell research: assessing the accuracy of generated references - a preliminary study. Ann Med Surg (Lond). 2023;85(10):5275-5278. doi:10.1097/MS9.0000000000001228. DOI: https://doi.org/10.1097/MS9.0000000000001228
Athaluri SA, Manthena SV, Kesapragada VSRKM, Yarlagadda V, Dave T, Duddumpudi RTS. Exploring the Boundaries of Reality: Investigating the Phenomenon of Artificial Intelligence Hallucination in Scientific Writing Through ChatGPT References. Cureus. 2023;15(4):e37432. doi:10.7759/cureus.37432. DOI: https://doi.org/10.7759/cureus.37432
Kwong JCC, Khondker A, Lajkosz K, et al. APPRAISE-AI Tool for Quantitative Evaluation of AI Studies for Clinical Decision Support. JAMA Netw Open. 2023;6(9):e2335377. doi:10.1001/jamanetworkopen.2023.35377. DOI: https://doi.org/10.1001/jamanetworkopen.2023.35377
Antoniou T, Mamdani M. Evaluation of machine learning solutions in medicine. CMAJ. 2021;193(36):E1425-E1429. doi:10.1503/cmaj.210036. DOI: https://doi.org/10.1503/cmaj.210036
Rusinovich Y, Rusinovich V. Do You Consent to the Use of Your Biological Data for Training ML and AI Models? Online Survey Targeting Clinicians and Researchers. Web3MLHS. 2024;1(1). doi:10.62487/yyx99243. DOI: https://doi.org/10.62487/yyx99243
Aggarwal R, Farag S, Martin G, Ashrafian H, Darzi A. Patient Perceptions on Data Sharing and Applying Artificial Intelligence to Health Care Data: Cross-sectional Survey. J Med Internet Res. 2021;23(8):e26162. doi:10.2196/26162. DOI: https://doi.org/10.2196/26162
Moorcraft SY, Marriott C, Peckitt C, et al. Patients' willingness to participate in clinical trials and their views on aspects of cancer research: results of a prospective patient survey. Trials. 2016;17:17. doi:10.1186/s13063-015-1105-3. DOI: https://doi.org/10.1186/s13063-015-1105-3
Parry MW, Markowitz JS, Nordberg CM, Patel A, Bronson WH, DelSole EM. Patient Perspectives on Artificial Intelligence in Healthcare Decision Making: A Multi-Center Comparative Study. Indian J Orthop. 2023;57(5):653-665. doi:10.1007/s43465-023-00845-2. DOI: https://doi.org/10.1007/s43465-023-00845-2
Richardson JP, Smith C, Curtis S, et al. Patient apprehensions about the use of artificial intelligence in healthcare. NPJ Digit Med. 2021;4(1):140. doi:10.1038/s41746-021-00509-1. DOI: https://doi.org/10.1038/s41746-021-00509-1
Rusinovich Y, Rusinovich V. Confounders in Predictive Medical Models: The Role of Religion. Web3MLHS. 2024;1(1). doi:10.62487/2rm68r13. DOI: https://doi.org/10.62487/2rm68r13
Rusinovich Y, Rusinovich V. Confounders in Predictive Medical Models: Roles of Nationality and Immigrant Status. Web3MLHS. 2024;1(1). doi:10.62487/vc54ms96. DOI: https://doi.org/10.62487/vc54ms96
Agency for Healthcare Research and Quality. Impact of Healthcare Algorithms on Racial and Ethnic Disparities in Health and Healthcare. https://effectivehealthcare.ahrq.gov/sites/default/files/related_files/cer-268-racial-disparities-health-healthcare.pdf.
Schlundt DG, Franklin MD, Patel K, et al. Religious affiliation, health behaviors and outcomes: Nashville REACH 2010. Am J Health Behav. 2008;32(6):714-724. doi:10.5555/ajhb.2008.32.6.714. DOI: https://doi.org/10.5993/AJHB.32.6.15
Cary MP, Zink A, Wei S, et al. Mitigating Racial And Ethnic Bias And Advancing Health Equity In Clinical Algorithms: A Scoping Review. Health Aff (Millwood). 2023;42(10):1359-1368. doi:10.1377/hlthaff.2023.00553. DOI: https://doi.org/10.1377/hlthaff.2023.00553
Shanklin R, Samorani M, Harris S, Santoro MA. Ethical Redress of Racial Inequities in AI: Lessons from Decoupling Machine Learning from Optimization in Medical Appointment Scheduling. Philos Technol. 2022;35(4):96. doi:10.1007/s13347-022-00590-8. DOI: https://doi.org/10.1007/s13347-022-00590-8
Allen A, Mataraso S, Siefkas A, et al. A Racially Unbiased, Machine Learning Approach to Prediction of Mortality: Algorithm Development Study. JMIR Public Health Surveill. 2020;6(4):e22400. doi:10.2196/22400. DOI: https://doi.org/10.2196/22400
Andrade C. Confounding. Indian J Psychiatry. 2007;49(2):129-131. doi:10.4103/0019-5545.33263. DOI: https://doi.org/10.4103/0019-5545.33263
Alsubaie MK, Dolezal M, Sheikh IS, et al. Religious coping, perceived discrimination, and posttraumatic growth in an international sample of forcibly displaced Muslims. Ment Health Relig Cult. 2021;24(9):976-992. doi:10.1080/13674676.2021.1973978. DOI: https://doi.org/10.1080/13674676.2021.1973978
Scheitle CP, Frost J, Ecklund EH. The Association between Religious Discrimination and Health: Disaggregating by Types of Discrimination Experiences, Religious Tradition, and Forms of Health. Scientific Study of Religion. 2023;62(4):845-868. doi:10.1111/jssr.12871. DOI: https://doi.org/10.1111/jssr.12871
Jordanova V, Crawford MJ, McManus S, Bebbington P, Brugha T. Religious discrimination and common mental disorders in England: a nationally representative population-based study. Soc Psychiatry Psychiatr Epi-demiol. 2015;50(11):1723-1729. doi:10.1007/s00127-015-1110-6. DOI: https://doi.org/10.1007/s00127-015-1110-6
Baqai B, Azam L, Davila O, Murrar S, Padela AI. Religious Identity Discrimination in the Physician Workforce: Insights from Two National Studies of Muslim Clinicians in the US. J Gen Intern Med. 2023;38(5):1167-1174. doi:10.1007/s11606-022-07923-5. DOI: https://doi.org/10.1007/s11606-022-07923-5
Bender M, van Osch Y, He J, Güngör D, Eldja A. The role of perceived discrimination in linking religious practices and well-being: A study among Muslim Afghan refugees in the Netherlands. Int J Psychol. 2022;57(4):445-455. doi:10.1002/ijop.12854. DOI: https://doi.org/10.1002/ijop.12854
Wu Z, Schimmele CM. Perceived religious discrimination and mental health. Ethn Health. 2021;26(7):963-980. doi:10.1080/13557858.2019.1620176. DOI: https://doi.org/10.1080/13557858.2019.1620176
Sharif MZ, Truong M, Alam O, et al. The association between experiences of religious discrimination, social-emotional and sleep outcomes among youth in Australia. SSM Popul Health. 2021;15:100883. doi:10.1016/j.ssmph.2021.100883. DOI: https://doi.org/10.1016/j.ssmph.2021.100883
An introduction to the UNESCO Recommendation on Open Science; 2022.
Shneiderman B. Human-Centered AI: Oxford University PressOxford; 2022. DOI: https://doi.org/10.1093/oso/9780192845290.001.0001
Gholamzadeh Nikjoo R, Partovi Y, Joudyian N. Involvement of charities in Iran's health care system: a qualitative study on problems and executive/legal/supportive requirements. BMC Health Serv Res. 2021;21(1):181. doi:10.1186/s12913-021-06187-9. DOI: https://doi.org/10.1186/s12913-021-06187-9
Tu HT, Reschovsky JD. Assessments of medical care by enrollees in for-profit and nonprofit health maintenance organizations. N Engl J Med. 2002;346(17):1288-1293. doi:10.1056/NEJMsa011250. DOI: https://doi.org/10.1056/NEJMsa011250
Schlesinger M, Quon N, Wynia M, Cummins D, Gray B. Profit-seeking, corporate control, and the trustworthiness of health care organizations: assessments of health plan performance by their affiliated physicians. Health Serv Res. 2005;40(3):605-645. doi:10.1111/j.1475-6773.2005.00377.x. DOI: https://doi.org/10.1111/j.1475-6773.2005.00377.x
Zare H, Eisenberg M, Anderson G. Charity Care and Community Benefit in Non-Profit Hospitals: Definition and Requirements. Inquiry. 2021;58:469580211028180. doi:10.1177/00469580211028180. DOI: https://doi.org/10.1177/00469580211028180
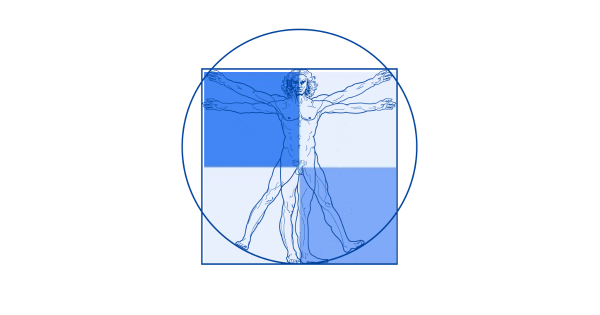
Downloads
Published
Issue
Section
License
Copyright (c) 2024 Yury Rusinovich (Author)

This work is licensed under a Creative Commons Attribution-ShareAlike 4.0 International License.
Add a Comment:
Comments:
Article views: 0