Impact of Fine-Tuned Machine Translation on Medical Documentation Translation and Client Satisfaction
DOI:
https://doi.org/10.62487/m0brp535Keywords:
Fine-Tuned Machine Translation (FTMT), Machine Translation Post-Editing (MTPE), ML and AI in medical translation, Bilingual Evaluation Understudy (BLEU)Abstract
Aim: The aim of this study was to assess the impact of Fine-Tuned Machine Translation (FTMT) models, followed by Machine Translation Post-Editing (MTPE), on the translation quality and client satisfaction in medical documentation. Materials and Methods: The research analyzed 733,632 words across 317 projects completed in 2023 by Lingrowth, a medical and life science translation service provider. These projects involved translations from 16 source languages to 34 target languages. Document types included Instructions for Use (IFU), Investigator Brochures (IB), Summary of Product Characteristics (SmPC), Clinical Study Reports (CSR), and Informed Consent Forms (ICF). The projects were categorized into two groups for comparison: translations performed by human translators using Translation Memory (TM) and those processed by FTMT followed by MTPE. The primary metrics for evaluation were the number of post-project finalization requests and instances of severe negative feedback from clients. Results: It was observed that human-translated projects had a higher frequency of post-project finalization requests, whereas MTPE projects after FTMT exhibited a marginally higher rate of severe negative feedback. However, statistical analysis indicated that these differences were not significant, suggesting that the introduction of FTMT models with subsequent MTPE does not adversely affect the overall quality of medical document translations. Conclusion: The study concludes that FTMT models, when supplemented by MTPE, are com-parable in effectiveness to traditional human translations in the context of medical documentation. This highlights the potential of integrating FTMT in translation workflows without compromising translation quality or client satisfaction.
Background
Introduction to machine translation in medical documentation, a synergy of ML and AI
The landscape of medical documentation translation has been profoundly reshaped by the integration of Machine Translation (MT), a transformation underpinned by advancements in Machine Learning (ML) and Artificial Intelligence (AI). Initially reliant on human translators for accuracy and medical expertise, the field has steadily pivoted towards technological solutions to meet the growing demand for swift and efficient translation services1. The inception of MT marked a pivotal shift, with early models primarily based on rule-based and statistical methods. However, the true game-changer has been the introduction of ML and AI into MT systems. These technologies have enabled MT to evolve from simple, literal translation algorithms to more sophisticated systems capable of understanding and interpreting the nuances of language, particularly in specialized fields like medicine2.
The landscape of medical documentation translation has been profoundly reshaped by the integration of Machine Translation (MT), a transformation underpinned by advancements in Machine Learning (ML) and Artificial Intelligence (AI). Initially reliant on human translators for accuracy and medical expertise, the field has steadily pivoted towards technological solutions to meet the growing demand for swift and efficient translation services1. The inception of MT marked a pivotal shift, with early models primarily based on rule-based and statistical methods. However, the true game-changer has been the introduction of ML and AI into MT systems. These technologies have enabled MT to evolve from simple, literal translation algorithms to more sophisticated systems capable of understanding and interpreting the nuances of language, particularly in specialized fields like medicine2.
Importance of translation Accuracy and client Satisfaction in medical fields
In the context of medical documentation, the stakes for translation accuracy are exceptionally high. Errors or ambiguities in translated medical texts, such as patient records, instruction manuals for medical devices, and pharmaceutical guidelines, can lead to severe consequences, including misdiagnoses and improper use of medical devices. Therefore, the accuracy of translations is not just a matter of linguistic correctness but also of patient safety and legal compliance3. Equally important is client satisfaction, which in the medical field often translates to the trust and confidence of healthcare providers, patients, and regulatory bodies in the translated materials4. High-quality translations enhance understanding among diverse populations, facilitate international collaboration in healthcare, and help in the dissemination of medical knowledge across linguistic barriers5.
Introduction to the concept of Fine-Tuned Machine Translation (FTMT) models
The latest innovation addressing the limitations of conventional MT is the development of Fine-Tuned Machine Translation (FTMT) models6. FTMT represents a significant leap from traditional MT approaches by integrating advanced machine learning techniques, particularly those in the realm of neural machine translation (NMT)7. NMT uses deep learning algorithms to not only translate words or phrases but to understand the context and semantics of the source language, thereby producing more accurate and natural translations. FTMT models take this a step further by being specially calibrated for specific domains, such as medical documentation. They are 'fine-tuned' using domain-specific corpora to understand and accurately translate the nuanced and technical language of medical texts8. This fine-tuning process is a collaborative endeavor involving AI, ML, and human expertise, creating a symbiotic relationship that harnesses the efficiency of technology and the nuanced understanding of human translators9.
Aim
This study aimed to investigate the impact of FTMT models on the quality of medical document translations and client satisfaction, measured by the number of negative client feedback or document finalization requests.
Material and Methods
Description of the data set
This study meticulously analyzed a series of 317 translation projects completed in the year 2023 by Lingrowth, a medical and life science translation service provider based in Aurora, US. These projects encompassed a diverse range of languages, involving 16 source languages and 34 target languages, thereby covering a broad linguistic spectrum. The subject matter areas were specifically focused on specialized fields within the medical domain, including medical devices, life sciences, clinical trial studies, and pharmaceuticals. In addition to the diversity in languages and subject matters, the types of documents translated were varied and crucial to the medical field. The document types included Instructions for Use (IFU), Investigator Brochures (IB), Summary of Product Characteristics (SmPC), Clinical Study Reports (CSR), Informed Consent Forms (ICF), among others. A crucial aspect of this study was the training of Machine Translation (MT) models tailored for each language pair and respective subject matter. This customization was fundamental in ensuring the relevancy and accuracy of the translations provided.
To evaluate the performance of the MT models, an automatic evaluation was applied, using the Bilingual Evaluation Understudy (BLEU) score as a metric. The BLEU scores for these models varied from 44.58 to 58.94, with an average score of 51.76. This variation in scores provided a quantitative measure of the translation quality across different language pairs and subject matters, serving as a baseline for comparing the effectiveness of Fine-Tuned Machine Translation (FTMT) models.
Methodology for evaluating the impact of FTMT
The evaluation methodology was bifurcated into two distinct categories to assess the impact of FTMT comprehensively:
1. Human Translations Using Translation Memory (TM): This category comprised projects where translations were primarily carried out by human translators utilizing Computer-Assisted Translation (CAT) tools and leveraging existing Translation Memories. This approach represents the traditional method of translation in professional settings.
2. Translations Post Machine Translation Editing (MTPE) After FTMT: The second category included projects where the initial translation was performed by FTMT models, followed by post-machine translation editing (MTPE). This method reflects a more modern approach, integrating advanced machine translation technologies.
The key metrics for evaluation in both categories were:
- Number of Client Requests for Additional Post-Project Finalization: This metric provided insight into the clients' satisfaction with the delivered translations, indicating how often clients felt the need for further refinement or clarification in the translated documents.
- Number of Severe Negative Quality Feedback: This measure was crucial for assessing the quality of translations. It involved counting instances where clients provided significantly negative feedback regarding the accuracy, readability, or overall quality of the translations.
By contrasting these metrics between the two categories of translation projects, this study aimed to draw comprehensive insights into the effectiveness of FTMT models in enhancing both the quality of medical document translations and the overall client satisfaction.
Results
Data Presentation: translated words, project types, and client feedback
The study analyzed a total of 733,632 words translated across 317 projects. These projects were divided into two main categories: Human Translations using Translation Memory (TM) in Computer-Assisted Translation (CAT) tools, and Machine Translation Post-Editing (MTPE) projects following the use of Fine-Tuned Machine Translation (FTMT) models. The distribution and feedback for these categories were as follows:
- Human Translation Projects with TM: Comprised 189 projects, translating a total of 458,409 words. Within this category, there were 10 instances of additional post-project finalization requests from clients and 1 instance of severe negative quality feedback.
- MTPE Projects after FTMT: Encompassed 128 projects, translating a total of 275,223 words. This category experienced 2 additional post-project finalization requests from clients and 2 instances of severe negative quality feedback.
Comparative Analysis of Outcomes
The comparative analysis between human translation projects and MTPE projects after FTMT yielded the following insights:
Post-Project Finalization Requests:
- Human Translation Projects: 10 requests out of 189 projects (5.29%).
- MTPE Projects after FTMT: 2 requests out of 128 projects (1.56%).
This indicates that human translation projects experienced a higher rate of additional post-project finalization requests compared to MTPE projects.
Severe Negative Quality Feedback:
- Human Translation Projects: 1 feedback instance out of 189 projects (0.53%)
- MTPE Projects after FTMT: 2 feedback instances out of 128 projects (1.56%)
The rate of severe negative feedback was higher in the MTPE projects after FTMT compared to human translation projects.
The chi-square tests suggest that while there is a trend towards more post-project finalization requests in human translation projects, this difference is not statistically significant (p = 0.094). Similarly, the difference in severe negative feedback rates does not reach statistical significance (p = 0.355).
These results suggest that while there are observable differences in client feed-back between the two types of translation projects, these differences are not statistically significant. This indicates that both human translations and FTMT can be effective methods, each with its own strengths and weaknesses in the context of translating medical documentation.
Discussion
Interpretation of findings
The study's findings offer a nuanced perspective on the role of Fine-Tuned Machine Translation (FTMT) models in medical document translation, especially when followed by Machine Translation Post-Editing (MTPE). Key observations include:
Client requests for post-project finalization: The higher rate of post-project finalization requests in human-translated projects, as compared to MTPE projects after FTMT, suggests that human translation is not immune to the need for further refinements. This may reflect the subjective nature of translation quality or specific client preferences that are not fully addressed by either translation method.
Severe negative quality feedback: The slightly increased incidence of severe negative feedback in MTPE projects after FTMT might point to certain limitations in these models' ability to fully capture the intricacies of medical language or specific client terminologies. However, the lack of statistical significance in this difference indicates that the quality of translations produced by FTMT models, followed by MTPE, is almost on par with that of human translations.
Impact of FTMT models on translation quality: Crucially, the study reveals that the utilization of FTMT models, complemented by MTPE, does not significantly impact the overall quality of translations compared to traditional human translations. This finding suggests that while FTMT models are not necessarily superior, their integration into the translation workflow does not com-promise quality. It underscores the potential of FTMT models as a supportive tool in the translation process, especially in scenarios where efficiency and scalability are essential.
Limitations
The study's reliance on client feedback as the primary metric for assessing translation quality, while valuable, might not capture the full spectrum of translation accuracy. The scope of the study, limited to projects from a single year and specific language pairs and document types, could affect the broader applicability of the findings.
Moreover, a significant limitation lies in the method of evaluating the FTMT models, which were assessed using machine evaluation techniques. While these methods are efficient and provide quantitative data, they lack the nuanced understanding that a linguist's evaluation could offer. For a more comprehensive and objective assessment of translation quality, incorporating evaluations by professional linguists alongside machine evaluations would be beneficial. This dual approach could provide a more rounded understanding of the translation quality, encompassing both the technical accuracy and the contextual appropriateness of the translations.
Conclusion
This study set out to assess the impact of Fine-Tuned Machine Translation models, followed by Machine Translation Post-Editing, on the translation of medical documentation. The results suggest that the use of FTMT models, coupled with subsequent MTPE, has negligible impact on the overall quality of translations when compared to human translations. The differences in client satisfaction and quality feedback between the two approaches were not statistically significant, indicating that FTMT models, when used in conjunction with MTPE, are an effective method in the medical translation workflow.
Future research should consider a wider range of languages and document types and incorporate more objective measures of translation accuracy. Enhancing the FTMT models based on specific feedback areas could further optimize their effectiveness in medical documentation translation. This study contributes valuable insights into the evolving landscape of translation technologies, high-lighting the potential of integrating advanced machine learning and AI in this field.
Conflict of Interest: AV states that no conflict of interest exists.
Authorship: AV: concept, data analysis, original draft, review, and editing.
References
1 Fernandez-Moure JS. Lost in Translation: The Gap in Scientific Advancements and Clinical Application. Front Bioeng Biotechnol. 2016;4:43. doi:10.3389/fbioe.2016.00043.
2 Terranova N, Venkatakrishnan K, Benincosa LJ. Application of Machine Learning in Translational Medicine: Current Status and Future Opportunities. AAPS J. 2021;23(4):74. doi:10.1208/s12248-021-00593-x.
3 Abdelrahman W, Abdelmageed A. Medical record keeping: clarity, accuracy, and timeliness are essential. BMJ. 2014:f7716. doi:10.1136/bmj.f7716.
4 Asan O, Yu Z, Crotty BH. How clinician-patient communication affects trust in health information sources: Temporal trends from a national cross-sectional survey. PLoS One. 2021;16(2):e0247583. doi:10.1371/journal.pone.0247583.
5 Garcia-Castillo D, Fetters MD. Quality in medical translations: a review. J Health Care Poor Underserved. 2007;18(1):74-84. doi:10.1353/hpu.2007.0009.
6 Unanue IJ, Parnell J, Piccardi M. BERTTune: Fine-Tuning Neural Machine Translation with BERTScore; 2021.
8 Pampari A, Raghavan P, Liang J, Peng J. emrQA: A Large Corpus for Question Answering on Electronic Medical Records. In: Riloff E, Chiang D, Hockenmaier J, Tsujii J, eds. Proceedings of the 2018 Conference on Empirical Methods in Natural Language Processing. Stroudsburg, PA, USA: Association for Computational Linguistics; 2018:2357-2368.
References
Fernandez-Moure JS (2016). Lost in Translation: The Gap in Scientific Advancements and Clinical Application. Frontiers in Bioengineering and Biotechnology, 4:43. doi:10.3389/fbioe.2016.00043. DOI: https://doi.org/10.3389/fbioe.2016.00043
Terranova N., Venkatakrishnan K., Benincosa L.J. (2021). Application of Machine Learning in Translational Medicine: Current Status and Future Opportunities. The AAPS Journal. 24(1):19. doi:10.1208/s12248-021-00593-x. DOI: https://doi.org/10.1208/s12248-021-00593-x
Abdelrahman W, Abdelmageed A. (2014). Medical record keeping: clarity, accuracy, and timeliness are essential. BMJ. 348:f7716. doi:10.1136/bmj.f7716. DOI: https://doi.org/10.1136/bmj.f7716
Asan O, Yu Z, Crotty BH. (2021). How clinician-patient communication affects trust in health information sources: Temporal trends from a national cross-sectional survey. PLOS ONE. doi:10.1371/journal.pone.0247583. DOI: https://doi.org/10.1371/journal.pone.0247583
Garcia-Castillo D, Fetters MD. (2007). Quality in medical translations: a review. Journal of Health Care for the Poor and Underserved. 18(1):74-84. doi:10.1353/hpu.2007.0009. DOI: https://doi.org/10.1353/hpu.2007.0009
Jauregi Unanue I. (2021). BERTTune: Fine-Tuning Neural Machine Translation with BERTScore. arXiv. [2106.02208]. doi:10.48550/arXiv.2106.02208. DOI: https://doi.org/10.18653/v1/2021.acl-short.115
Miceli Barone AV, Haddow B, Germann U, Sennrich R. (2017). Regularization techniques for fine-tuning in neural machine translation. In Proceedings of the 2017 Conference on Empirical Methods in Natural Language Processing, pages 1489–1494, Copenhagen, Denmark. Association for Computational Linguistics. doi:10.18653/v1/D17-1156. DOI: https://doi.org/10.18653/v1/D17-1156
Yang, X., Chen, A., Harle, C. A., Hogan, W. R., Shenkman, E. A., Bian, J., & Wu, Y. (2018). emrQA: A Large Corpus for Question Answering on Electronic Medical Records. Proceedings of the 2018 Conference on Empirical Methods in Natural Language Processing. 2357–2368. doi:10.18653/v1/D18-1258. DOI: https://doi.org/10.18653/v1/D18-1258
Baziotis, C., Haddow, B., & Birch, A. (2020). Language Model Prior for Low-Resource Neural Machine Translation. In Proceedings of the Conference on Empirical Methods for Natural Language Processing. 7622–7634. doi:10.18653/v1/2020.emnlp-main.615. DOI: https://doi.org/10.18653/v1/2020.emnlp-main.615
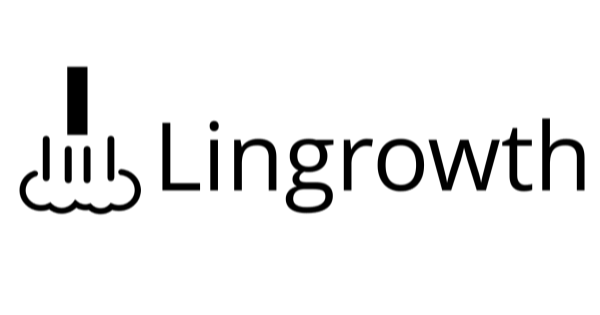
Downloads
Published
Issue
Section
License
Copyright (c) 2024 Alexander Vareiko (Author)

This work is licensed under a Creative Commons Attribution-ShareAlike 4.0 International License.
Add a Comment:
Comments:
Article views: 0